AI and Risk Management: Opportunities and Challenges in the Financial Sector
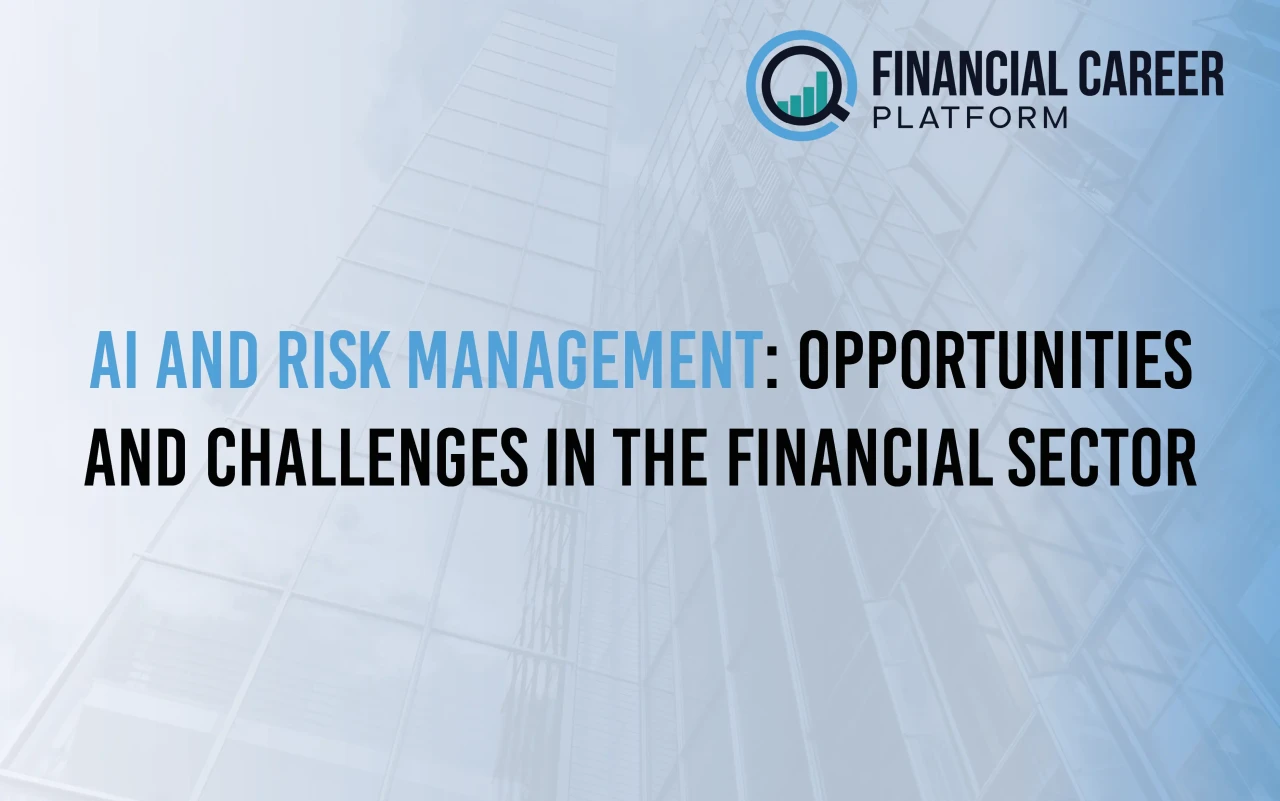
The rise of Artificial Intelligence (AI) within the financial sector has increased exponentially in recent years, and one of the many fields that AI has significantly impacted is risk management and assessment, where financial companies were often required to process large amounts of historical data to infer future outcomes. AI can be regarded as a catalyst, a tool to accelerate the processing of data, and with the emergence of new types of AI, such as Generative AI, which produces, among other things, text resembling human interactions, its use is becoming increasingly more accessible.
Benefits and Applications of AI and Machine learning in Risk Management
Although it is difficult to list all the possible applications of AI in risk management processes, auditing and consulting companies such as McKinsey, EY, and KPMG have pinpointed particularly popular/interesting ones that have been listed hereunder. Here, it is important to underline that although for some, it might be sufficient, many activities require human input to maximise the use of AI.
Risk analysis and credit modelling: Although banks may still refer to traditional risk models, AI and Machine Learning (ML) can be used to optimise parameters and variable selection processes. Here, AI can also be used as a sparring companion by risk analysts so that they can brainstorm and take into consideration more variables.
Fraud detection and risk identification: Here, AI and ML have a more independent role, as the vast amount of historical data of credit card transactions, for example, makes it easier to assess the likelihood of credit card fraud.
Trading: Similarly to fraud prevention, crimes such as insider trading and market manipulation can often be flagged by analysing traders’ behaviour with AI and using historical data to identify possible trader misconduct.
Climate risk: AI can automate data collection for counterparty transition risk assessments, signal early warnings based on events, and even support with reports for environmental, social, and governance (ESG) metrics.
Risks of AI and ML
As with many emerging technologies, the benefits of AI and ML are countered by many risks and drawbacks, which underline the importance of maintaining human supervision/intervention when they are used. Among others, risks can be:
Algorithmic biases: Because AI and ML are based on historical data and build decisions based on identified patterns if the data they are fed contains biases, then the outcomes they produce will be biased too. If we pair this with an overestimation of what can be accomplished by them, we end up with jeopardized outcomes and/or poor quality.
Programming errors: AI and ML can rely on heavy programming that allows them to function the way they do. As such, mistakes in the code that back up their decision-making processes will result in faulty results.
Processing of Personal Data: Regulations to increase data protection has increased significantly over the years, with the General Data Protection Regulation—part of European legislation—holding one of the highest standards in this regard. Because of the vast amount of data needed by AI and ML, personal data is often processed by them, which poses a risk to the right of privacy of the people whose data is being used, especially when automated decision-making is involved.
Cybersecurity: Because AI and ML can be involved in the processing of the personal data of customers, they can often become targets of hacker attacks.
Considerations for the Future
Given the extensive risks carried by AI, management should ensure that they are ready for AI before integrating it into their systems and ask themselves:
Do we fully understand the impact of AI on our organization’s business model, stakeholders, culture, and strategy?
The adoption of new procedures also requires getting employees ready for a significant culture change and requires all risk professionals to be aware of AI capabilities and limitations. As AI advances, its responsible integration into risk management will depend not only on technological advancement but also on the human factors guiding it.
Bibliography
- EY https://www.ey.com/en_it/insights/assurance/why-ai-is-both-a-risk-and-a-way-to-manage-risk.
- KPMG https://kpmg.com/ae/en/home/insights/2021/09/artificial-intelligence-in-risk-management.html.
- ECB https://www.ecb.europa.eu/press/financial-stability-publications/fsr/special/html/ecb.fsrart202405_02~58c3ce5246.en.html.
- PWC https://www.pwc.com/us/en/industries/financial-services/library/gen-ai-and-risk-management.html.
- McKinsey https://www.mckinsey.com/capabilities/risk-and-resilience/our-insights/how-generative-ai-can-help-banks-manage-risk-and-compliance.